P-Values Part 3: Interpretation in Context
My previous two posts laid out the fundamental need and concept behind the p-value and the statistical terms required to discuss it. However, before we dive into how exactly people use p-values correctly and incorrectly, I want to provide a broader overview of how p-values fit into scientific research.
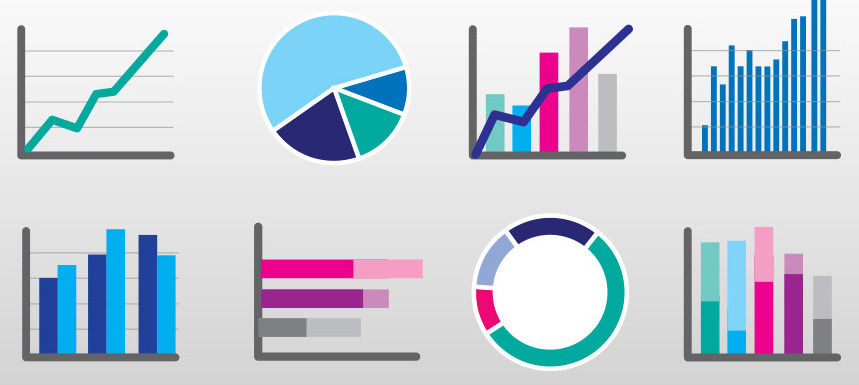
To do this, I will describe eight factors that should almost always be considered in addition to the p-value when interpreting the results of an experiment. I'm kinda skipping to the end here -- I could imagine this being the final blog entry in this series, rather than the third. But I'm emphasizing this content for a reason: more than any definition or nuance of a p-value, the proper consideration of these contextual factors will guide you to a reasonable interpretation of a statistical result.
1. Effect Size
When researchers ask a question -- like whether race can explain variation in life expectancy that other variables can't -- there are two very important kinds of numbers that they look at: the effect size, and the significance. So far, we've just been talking about the latter. The effect size is, thank god, way easier to understand. It would be something like this: African Americans are found to have a life expectancy on average 2.8 years shorter than white Americans, after controlling for other variables. Then, that particular finding would also have a p-value. It could be 0.34 (indicating the effect is not statistically significant) or 0.0009 (indicating that it is) or whatever. Note that effect sizes can be calculated a few different ways, including via the direct statistical comparison of populations or as the coefficients produced by a regression. Also note that while the p-value is a probability that always exists in the range from 0 to 1, effect sizes don't always have identical interpretations. Sometimes they show the effect that a unit change in an explanatory variable (e.g. race) has on the the variable of interest (e.g. life expectancy), while in other cases they assume a percentage change in that explanatory variable instead. Furthermore, it's important to identify if the effect is measured with exactly the same units variable of interest, and not the standard deviation, or some other unit. These distinctions are critical for correctly interpreting an effect size.
Regardless of those details however, the effect size is the scientific finding. The associated p-value then indicates how robust that finding is to expected noise in the data. Therefore, it's the combination of these two that makes a result compelling: a reasonably large effect that is also statistically significant. Oftentimes, they go together -- the larger the effect is, more robust it will typically be against noise -- but not always. If you get a "good" p-value (e.g. <0.05) but the effect size is tiny, the p-value doesn't automatically make the finding noteworthy.
2. Data Collection
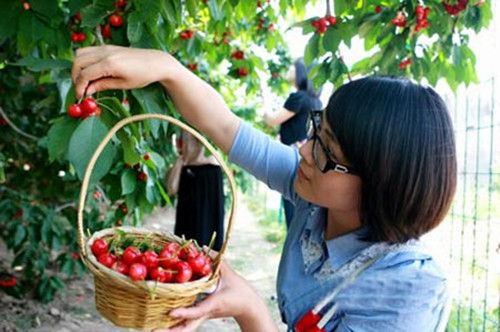
It matters a lot how you get your data. Unfortunately, it's often rather easy to design an experiment which is biased to produce the results you're looking for. Even worse, it's often difficult for others to recognize this practice from the outside. For example, if you're looking to show that bisexuality does not exist in men, and you advertise for volunteers in two types of locations -- those frequented specifically by straight men, and another set of places visited by mostly gay men -- you may easily find that indeed, there were remarkably few men in the overall sample that showed possible attraction to both genders. Here, the statistical test has been tricked. It expected a certain amount of baseline variation, which the experiment avoided capturing (intentionally or not). So, the results seem unlikely to come from the null hypothesis (which by default assumes that bisexuality exists for both genders), and you get a small p-value. However, that small p-value doesn't necessarily say that the real world looks unlike the null hypothesis. It may instead say simply that the experimental methodology was not well aligned to test the null the hypothesis in the first place.
3. Data Generation
A similar problem arises in studies that rely on Monte Carlo simulation -- and there are a lot of them. Monte Carlo just means that some system or process was simulated on a computer in a way that in included probabilities. You might recall that in the first post, I wrote about how the calculation of a p-value accounts for the benefit of more data, and therefore a larger sample size almost always produces a smaller p-value, and a more significant result. One challenge that Monte Carlo simulation presents is that you can set the sample size (often known as the number of replications) to almost anything you want. You're only limited by the computational capacity of your computer. In many cases, this means that you can simulate a process many more times than you would ever measure it in real life. The result can be artificially low p-values. It's not difficult -- or uncommon, unfortunately -- to make a questionable simulation but use it to produce "highly significant" results with p-values < 0.001. This happens because of the combination of overly large sample sizes and the fact that a simulation is always an approximation of reality. A simulation will never capture all the types of noise that you are exposed to in empirical experiments. Therefore, it's comparatively easy to produce a signal that can be separated from that minimal amount of baseline variation. So in some cases it's almost trivially easy to get significant results. And this can happen with well-made and poorly-made simulations alike. One way to confront this issue is to only run as many replications as actual cases in a row you expect would resemble the simulation, until something un-modeled would happen. In other words, if you've built a model for the operation of a proposed factory floor and each replication represents a day, how many actual days do you think would go by, on average, before something happened that's not in the model? Would you expect to go 10 days? 30? Or maybe just 3? Run only that many replications, because that's what you've actually built the simulation for. You can never construct a simulation that covers every possibility, and running hundreds or especially thousands of replications of a Monte Carlo simulation is often difficult to justify. Of course, this depends on how much complexity and uncertainty is included in the model vs left out.
4. Balancing Two Kinds of Risk
Just as experimental minutia matter, so does the big-picture research context. What kind of conclusion are you making; who are you going to share it with; what action would it recommend; and how bad is it if you make a mistake? Critically: is it worse to make a false discovery or to miss something? The answer depends on who you are, and what you're doing. A scientist desperately looking for an Ebola treatment while the epidemic rages in West Africa might be more afraid of not discovering an effective treatment than deploying something not helpful. In contrast, a policymaker looking to allocate funds might want to be very confident that what they're committing to is going to pan out and look good, even if potentially there's something better that gets overlooked in the process. The two have different risk preferences.
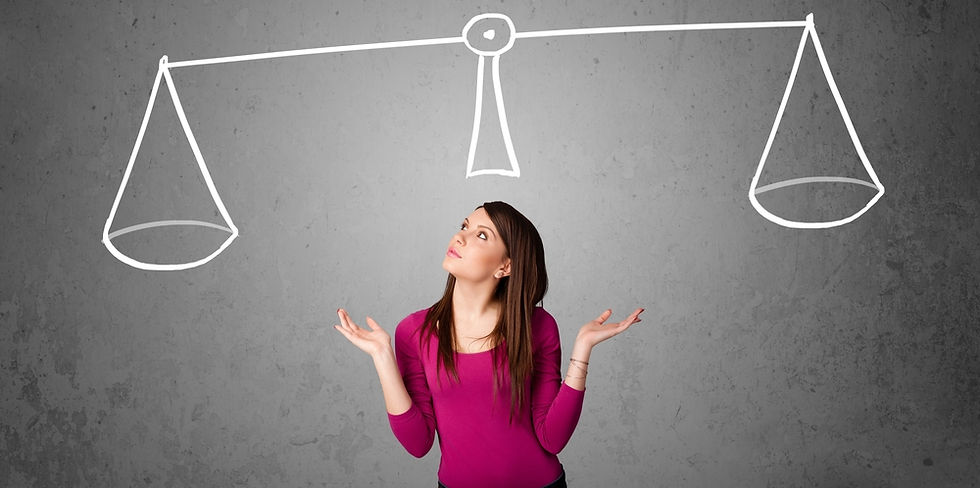
A Type I error occurs when the baseline model is rejected in favor of some notable scientific finding -- but that finding turns out to be wrong. Deploying an unhelpful Ebola treatment or funding an ineffective government program are examples of Type I error. A Type I error is a false discovery. P-values are related to the risk of a false discovery, because they tell us the rate at which the noise in the Ho would produce the evidence we have in front of us, as opposed to producing any other evidence. As described in the first two posts, a high p-value indicates that noise alone often produces results like those we found, and therefore it's doubtful that the Ebola treatment actually works, or that the government program is actually effective. We'd like to know the actual probability that the discovery is wrong, called the False Discovery Rate, but instead all we know is how often simple noise produces evidence like what we got. I discuss the False Discovery Rate in future blog posts in this series.
A Type II error represents the opposite problem. The baseline model was wrong -- something noteworthy was in fact going on! -- but we failed to reject Ho and therefore thought it was just noise. Examples of Type II error include missing the effective Ebola treatment, or overlooking the more effective government program. So a Type II error means you missed a real finding. P-values are not directly related to the risk of a Type II error, so we must do additional work to evaluate this risk. There will always be a balance in research -- and decision-making more broadly -- between Type I and Type II error. Between doing something wrong and failing to do something right. Between over-reacting and under-reacting. To strike the right balance, you must understand both the risk preferences at play in the scenario at hand and how to use the appropriate statistics.
5. Multiple Tests
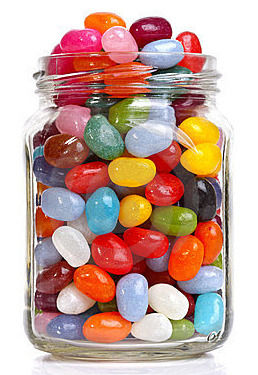
This issue reflects a kind of confirmation bias that has received increasing attention over the past several years. The basic problem is this: if you test for a lot of possible effects, it's more likely that you make a Type I error (a false discovery), simply because you have more opportunities to. For example, consider the hypothesis that jelly beans cause acne. Imagine a food science post-doc conducts a study and evaluates the results using the null hypothesis that jelly beans do not cause acne. He picks the common significance level of 0.05, below which a p-value will lead him to reject the null hypothesis. He finds that p = 0.39, and fails to reject the Ho. It seems entirely plausible that random noise produced the results he measured, and so he has no reason to believe that jelly beans cause acne. But the post-doc really needs to publish -- or maybe the cosmetics company funding the research really wants a positive result. So he reruns the data to see if maybe it's just one particular color of jelly bean that has the effect. Suppose there are ten colors, and he finds a set of p-values like this: 0.43, 0.92, 0.26, 0.59, 0.03, 0.88, 0.14, 0.35, 0.74, and 0.61 after testing each color individually. A responsible and statistically literate researcher would say that looks like a pile of null results, for an overall significance level of 0.05. But the post-doc pressed to publish might say "Look! p = 0.03 is less than 0.05! That's statistically significant evidence that green jelly beans cause acne!" If that had been the only hypothesis made, and the only one tested, then he would be correct. Instead, because he made 10 tests, he should have made a Bonferroni correction to the significance level, dividing it by the number of tests, to get 0.005 instead of 0.05. Using this proper significance level for individual tests would have yielded no statistically significant results.
However, most researchers know (or a colleague mentions) to adjust their significance level when it's that obvious. The real trouble often comes earlier in the research process and is widely discussed as p-hacking or data dredging. Imagine you just got a bunch of exclusive data. You immediately dig into it, looking for potential patterns. Many of your early leads don't hold up to further scrutiny, but you focus on those for which there appears to be continued evidence. You come up with theories to explain a few of the most compelling patterns. You experiment with a few ways to test these effects and make a few tweaks, excluding outliers here and there, and eventually settle on a formal statistical test. The result turns out to be statistically significant. Only a single p-value is produced (or published), but in reality, there were many tests conducted. This is a more pernicious version of the multiple tests problem, because it's so difficult to track. Recall the height-measuring example from the first post: was that an example of multiple tests? Repeated splits and adjustments to methodology are part of the scientific method -- but they also pose a serious statistical problem.
6. Bayesian Statistics
Bayesian statistics are always the elephant in the room when you talk about the limitations of p-values. Everything I've discussed thus far -- notably hypothesis testing and p-values -- belong to a world known as frequentist statistics. The differences between the two camps have been widely discussed. This blog post is not intended to provide a comprehensive overview of Bayesian methods. Nonetheless, Bayesian methods do provide an alternative approach to the same problem that motivates frequentist statistics and therefore deserve some attention. Frequentists view the world in terms of populations and totalities: if they were able to measure everything, they would calculate every parameter and effect perfectly. From that perspective, they work backwards to evaluate what their limited data might be able to say about the underlying truth. Bayesians on the other hand, assume that everything is probabilistic and changing, so all we can ever hope to do is estimate those probabilities and adjust them. They work forwards from educated guesses to estimated probabilities, and continuously update those estimates as more information comes in. Specifically, Bayes' Theorem is used to update the estimated probability of an event, given the information that you collect. These are two philosophies for approaching a single problem: we want to explain how the world works with firm rules, but there's a lot of variation when we actually make observations. Suppose that our health policy researcher is trying to decide whether route A or B gets her to work faster, on average. If she thinks like a frequentist she'd say "Well, if they were exactly equal, I know the distribution of results that would be theoretically produced for infinite trials. I'll take some measurements and see how they compare to that!" As a Bayesian, she'd say "Well, my experience is that route B is slightly faster. I'll make some measurements and adjust my thinking based on what I find." They are both credible approaches, and while many have defaulted to the frequentist view for a century or longer, Bayesian analyses are gaining popularity, in part due to faster computers. At a minimum, it's certainly worth considering when interpreting a frequentist result such as a p-value: how would a Bayesian view this?
7. Related Evidence
No experiment is done in a vacuum (or at least a perfect vacuum...). Science is inherently a distributed, self-monitoring, and evolving enterprise. It must be, because scientists strive to make discoveries and describe laws that are consistently true. Therefore, when a scientist makes a particular claim, they are really just making an addition to an evolving body of knowledge, measurements, theories, and questions.
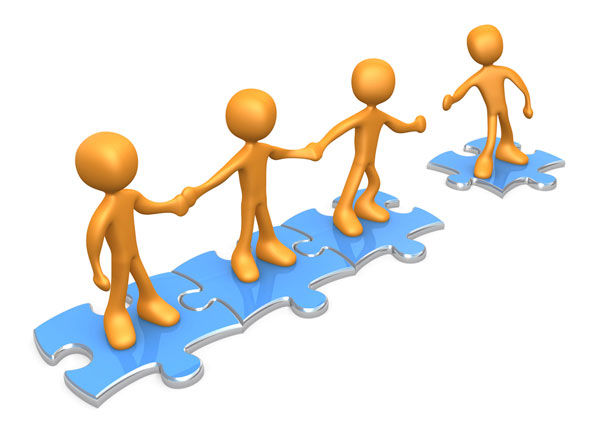
Consider a health policy researcher assessing the impact of the Affordable Care Act on healthcare costs. That's a hugely important and tremendously complex question. The researcher must pick a methodology with which to study the question, and it's going to be insufficient. But fortunately, she doesn't have to answer the question on her own. She has colleagues who might understand the issue differently. She knows of historical cases of attempted healthcare cost reduction in the U.S. and elsewhere, against which she can validate her analysis. She also is part of a much broader community of researchers investigating the same and related questions. Checking her work in these divergent ways helps her to avoid the temptation to view such complexity through the insufficient lens of a p-value.
8. Asking the Wrong Question
Referencing the traditional categories of Type I and Type II error, a buzzy phrase has been making the rounds: Type III error. As an evolving concept, it has competing definitions, and some statisticians today talk about not only Type III but also Type IV error. They are related concepts in that both Type III and IV error look at mistakes that can be made in research outside the extremely narrow activity of rejecting vs failing to reject a null hypothesis. A Type III error occurs when you reach the right answer, but for the wrong question.
Type I and Type II errors focus on how you use data to evaluate a particular hypothesis -- but that hypothesis was probably produced or informed by a larger model or theory. Furthermore, the results of the statistical test may well be used to then go back and confirm or adjust that larger model. This is a problem because Type I and Type II error say nothing about the larger model. Type III error is used therefore to point out that just because you find a statistically significant result with a hypothesis test, that does not necessarily mean that the result is relevant to the broader question or model that you are working with. In other words, you were asking the wrong question in the first place, in order to be able to make proper sense of what you then found in the data. Unfortunately, due to its nature, Type III error is quite difficult to estimate.
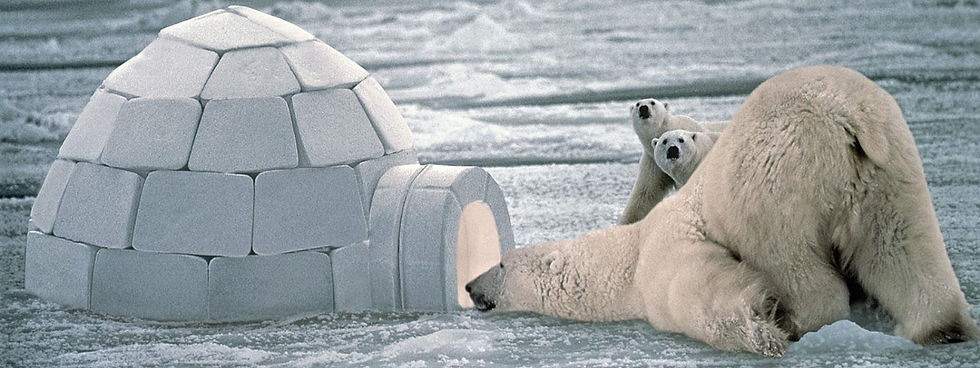
However, we can at least describe what it looks like, so that we're better equipped to avoid and identify it. The kinds of mistakes that generally lead to Type III errors include:
Poor theory development, such as ad-hoc additions or internal inconsistency
Operationalizing or measuring a variable improperly, compared to how it functions in the model
Selection of inappropriate null hypotheses
Improper formulation of causal architecture
That last one gets into the infamous correlation vs causation problem -- which is actually itself more complicated than typically described, but I won't get into that here. For a quick example of the basic point, imagine that a researcher is trying to explain the cause of babies' birth gender. He does a study and finds with high statistical significance that brighter and warmer clothes are strongly associated with the female gender. It's crazy to conclude from this result that the brighter clothing caused the babies to be female. Rather, the two traits are simply correlated. If anything the causality would go the other way. This is an example of an improperly formulated causal architecture.
Given that the researcher was collecting data on fashion preference, "what causes gender differentiation before birth?" was the wrong question to ask. Since babies don't wear clothes before birth, this mistake is pretty obvious. In practice, however, it's often much less obvious. Consider a scientific finding that indicates a strong connection between poor dental hygiene and homelessness. How would you incorporate that finding into a broader theory of homelessness? What is the right question -- the one for which that result provides an answer?
Much More Than a P-Value
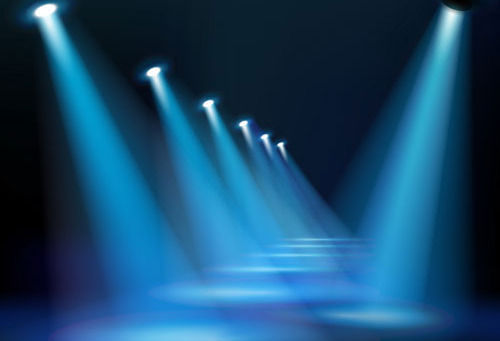
The mere fact that some data or statistics appear to point to a particular result does not make that result true. Making sense of statistical results is quite difficult when you consider all the various mistakes we can make. Maybe we were asking the wrong question. Or maybe we typed in a number wrong or read something backwards. Or maybe our experiment was flawed. Or maybe we aren't thinking about risks or effect sizes correctly. The list goes on. The best thing any researcher can do is to repeatedly cross-check results and interpretations through as many views as possible. Each of these views brings additional clarity to the topic at hand. P-values are just one of these many many ways to characterize scientific findings. In the next post, I return to p-values in order to contrast them with Bayesian statistics.